
AI-Driven Alert Triage with DHIS2 Transforms Disease Surveillance in Tanzania
Tanzania’s Ministry of Health has introduced an AI-based DHIS2 integration for event-based surveillance of outbreak-prone diseases, reducing average time to triage for the majority of submitted alerts from 36 hours to almost instantaneous.
Tanzania, a country of nearly 65 million people, faces persistent public health challenges due to its diverse geography, population distribution, and the prevalence of both communicable and noncommunicable diseases. While the country has made significant strides in strengthening its healthcare system, outbreaks of cholera, Marburg virus, anthrax, and other epidemic-prone diseases remain a serious concern, particularly in high-risk regions. Rapid detection and response to potential health threats are critical for effective disease control and prevention. Over the years, Tanzania’s Ministry of Health, with support from partners like the U.S. Centers for Disease Control and Prevention (CDC) and the World Health Organization (WHO), has worked to modernize its health information systems to improve surveillance, early detection, and timely intervention for public health threats.
In 2021, with support from the CDC, Tanzania’s Ministry of Health launched its electronic Integrated Disease Surveillance and Response (e-IDSR) system based on DHIS2 to alert health teams to possible outbreaks. This effort boosted data collection and enabled real-time reporting and analysis, significantly improving Tanzania’s ability to monitor and respond to disease outbreaks. By 2022, e-IDSR was fully implemented, and it proved crucial for Tanzania’s rapid detection and containment efforts for the Marburg Virus Disease outbreak in 2023. Building on this success, the MoH then introduced an event-based surveillance (EBS) system that complements the e-IDSR by collecting unstructured data from the community—such as SMS messages submitted by the public about potential disease cases (known as “rumors” or “alerts”)—into a program in DHIS2 where they can be triaged and flagged for follow-up. The EBS system offered several key disease surveillance enhancements, but manual triage of alerts quickly became a bottleneck in the system, due to the high volume of rumors submitted and the limited human resources to process them.
To address this challenge, the Ministry of Health, in collaboration with Tanzania’s University of Dar es Salaam (UDSM) DHIS2 Lab and its international partners, developed an AI-powered report triage system to enable more timely and efficient event-based surveillance. This innovation has significantly improved the speed and efficiency of processing these alerts, reducing the average triage time from 36 hours to almost instantaneous, and successfully processing 85% of previously untriaged alerts.

Transforming Public Health Surveillance in Tanzania
Event-based surveillance (EBS) is a proactive public health approach that includes collecting, analyzing, and interpreting health-related events from multiple sources, including SMS messages about suspected or observed disease cases, and reports by community health workers. The process involves five key steps: reporting, triage, verification, investigation, and action. Tanzania’s EBS system uses a DHIS2 Tracker program, where each incoming alert is registered automatically and can be digitally tracked and updated through each of these steps. Of these five steps, triage—the process of screening and prioritizing incoming alerts about potential health threats—was a critical challenge within Tanzania’s surveillance system, as staff struggled to effectively process the approximately 15,000 alerts submitted from 2023 to January 2024.
The AI-based triage initiative followed a structured development process. Requirement gathering began in May 2024, followed by algorithm development in June. The UDSM team completed system integration and testing in July, and deployment began soon after, with ongoing feedback collection to refine the system further. The system has now been deployed in five high-risk regions and has successfully processed 85% of the backlog of more than 15,000 reports, revealing that approximately 23% warranted further investigation.
Before the AI integration, the manual triage process often took 36 to 48 hours, delaying the identification of potential outbreaks. Additionally, in the five regions where the system was piloted, which had been selected because of their vulnerability to outbreaks such as cholera, Marburg virus, and anthrax, approximately 60% of alerts remained untriaged due to limited human resources. The reliance on manual intervention not only slowed outbreak detection but also could have allowed critical health threats to go unnoticed for extended periods. With the introduction of automated triage using AI-driven keyword filtering and duplicate detection, the system can now process incoming reports almost instantly. This has dramatically improved response times and allowed public health teams to identify and verify emerging health threats much more quickly.
Introducing AI for Smarter Disease Surveillance
The AI-powered triage system works by automating the classification of incoming reports. It begins by checking for duplicate submissions, reducing redundancy in the dataset. Then, keyword filtering is applied, using predefined disease names and symptoms to assess the relevance of each alert. If an alert meets specific case definitions, it is flagged for further verification. While the verification step—assessing an alert’s relevance for early warning and response—is still performed manually, the structured AI-driven approach to triage has streamlined the process significantly by allowing staff to focus on reviewing alerts that are more likely to be relevant.

By integrating this workflow into DHIS2, all health alerts pass through a structured triage process before being entered into Tanzania’s e-IDSR system. Reports can be submitted directly into DHIS2 via SMS by health workers or the general public, or through a custom mobile app or web portal that health workers have access to. Each alert is automatically assigned its own Tracked Entity Instance in DHIS2, allowing for seamless tracking through the entire surveillance process. If an event is triaged and confirmed to be valid, it transitions from EBS to the indicator-based surveillance system, ensuring a coordinated national response.
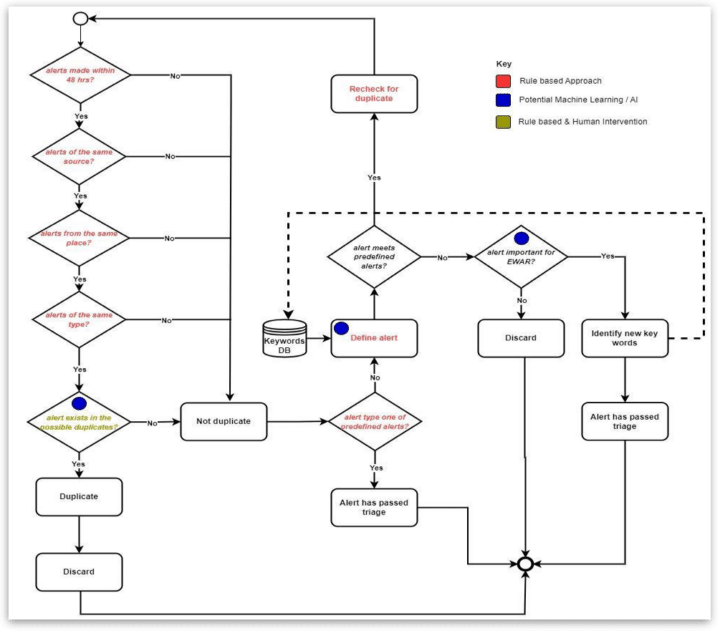
The Future of AI and DHIS2 in Public Health Surveillance
The successful integration of AI-based triage with DHIS2 marks a major step forward in strengthening Tanzania’s disease surveillance infrastructure. The system has already demonstrated significant improvements in efficiency and response time, and its continued evolution promises even greater impact. The team at the UDSM DHIS2 Lab have outlined the next steps in their implementation plan, including the introduction of a machine learning algorithm to enhance the triage tool’s capabilities.
In the future, the MoH also plans to include automated alerts from social media platforms, with an electronic tool to crawl public social media activity and create notifications about possible disease cases into the EBS system. Additionally, efforts are underway to expand the EBS system and triage tool deployment to the remaining 21 regions. By expanding the system’s reach, the UDSM team hopes to achieve a 95% auto-triage rate across all regions, further reducing the burden on human health workers and improving public health outcomes. There are also opportunities for linking the EBS and e-IDSR systems to other programs, such as immunization, which could support more effective planning of vaccination campaigns that target vaccine-preventable diseases.
This impact story is based on an abstract titled “Enhancing DHIS2 Event-Based Surveillance with AI,” submitted for the DHIS2 2025 Annual Conference, and an interview with the UDSM DHIS2 Lab team.